Invited Speaker
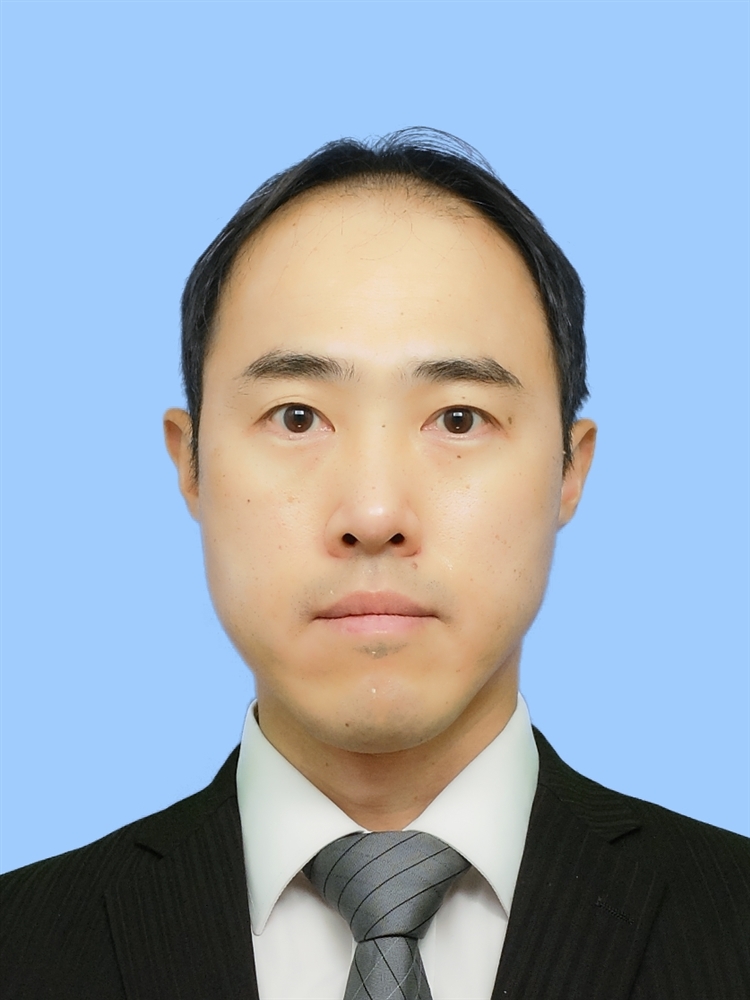
Hirotada Honda
Professor, Faculty of Information Networking for Innovation and Design (INIAD), Toyo University, JapanSpeech Title: On the Expressive Power of ODE-Based Neural Networks
Abstract: Ordinary differential equation (ODE)-based neural networks, such as the now well-known Neural ODE framework, have recently drawn increasing attention. These approaches treat a system of ODEs as a “continuous-depth” component within a neural network architecture. In this talk, I will explore the expressive power of ODE-based neural networks in a broad setting that includes manifold domains, shedding light on how continuous-time dynamics enrich their representational abilities. In addition, I will discuss learnability aspects from a statistical learning theory perspective, addressing questions of generalization and capacity. Time permitting, I will also consider the computational demands associated with training and evaluating these ODE-based models, highlighting both their potential advantages and practical challenges.
Biography: Hirotada Honda received a Ph.D. in Mathematics from Keio University in 2011. From 2002 to 2018, he worked at Nippon Telegraph and Telephone Corporation (NTT). Since 2018, he has been with Toyo University, where he is currently a Professor in the Faculty of Information Networking for Innovation and Design (INIAD). His research interests span statistical learning theory, mathematical optimization, and the application of differential tpology and differential equations to machine learning theory.